Solute carriers (SLCs) have often been overshadowed by more prominent protein families in drug discovery, such as kinases and G protein-coupled receptors. However, SLC proteins hold significant therapeutic potential due to their role in various diseases. Among them, the high-affinity norepinephrine transporter (NET/SLC6A2) has received considerable attention, but the limited chemical diversity of known ligands presents a challenge for identifying novel compounds. The new article published in Journal of Chemical Information and Modeling explores a computational screening pipeline developed to discover new NET inhibitors, utilizing a data-driven approach to expand known chemical space and optimize target selection.
Cracking the Code: Optimizing Proteochemometric Models
A novel approach was introduced to determine the ideal set of related proteins to include in a proteochemometric (PCM) model. This data-driven method improved performance over single target models by incorporating more data. The optimal number of related sequences included in the model hinged on the similarity, chemical diversity, and the number of data points per target, making the optimal amount data set-dependent.
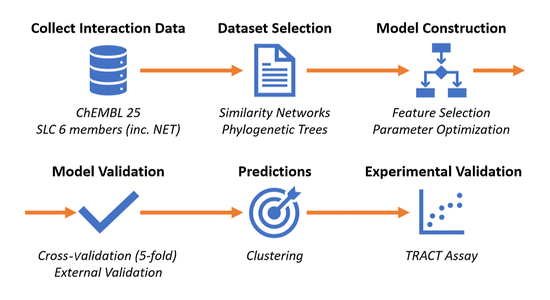
Figure above presents a schematic representation of the sequential computational steps employed in this study. The process began with data collection from ChEMBL 25, where interaction data for NET and related SLC6 members were obtained. To refine the data for relevance, both similarity networks (SNs) and phylogenetic trees were utilized, expanding the data set to include sequence information of related proteins and their corresponding chemical space. The model was then trained and optimized through feature selection and parameter optimization. Cross-validation was performed in conjunction with external validation from a separate test set (10% of the data), ensuring minimal overfitting. The optimized model was subsequently used to virtually screen the Enamine REAL database, and the top predicted 22,000 of the 600 million virtual compounds were clustered, yielding 46 chemically diverse candidates. Finally, cluster centers were selected for experimental validation using a TRACT assay.
Validating results
From the 46 diverse candidates, a subselection of 32 compounds was synthesized and subsequently tested using an impedance-based assay. Five hit compounds were identified (hit rate 16%) with sub-micromolar inhibitory potencies toward NET, demonstrating the potential of this data-driven approach to diversify known chemical space and identify novel ligands.
The five hit compounds not only exhibited sub-micromolar potencies toward NET, but also had an average Tanimoto similarity of 0.38 ± 0.12 to the training set, confirming their novelty. These scaffolds could serve as a launching pad for the design and synthesis of derivatives, quantitative structure-activity relationships, and subsequent hit optimizations of novel NET inhibitors.
This study showcases a really interesting data-driven approach to identify protein inhibitors by dynamically determining the optimal number of related proteins in a PCM setting. This method was successfully applied to identify novel NET inhibitors with similar affinities as the reference high-affinity NET inhibitor nisoxetine. The combination of machine learning models, optimized target selection, and virtual screening of compound databases has proven effective in discovering chemically diverse and potent NET inhibitors. This approach offers promise for delving into underexplored protein families like SLCs and holds the potential to significantly impact the pharmaceutical industry by broadening the chemical space of known ligands and unveiling new therapeutic candidates.
Read more about Enamine REAL compounds and learn how this technology can also improve your drug discovery project.